CausalXtract, a flexible pipeline to extract causal effects from live-cell time-lapse imaging data
Jan 17, 2025·,,,,,,,,,
Franck Simon
Maria Colomba Comes
Tiziana Tocci
Louise Dupuis
Vincent Cabeli
Nikita Lagrange
Arianna Mencattini
Maria Carla Parrini
Eugenio Martinelli
Hervé Isambert
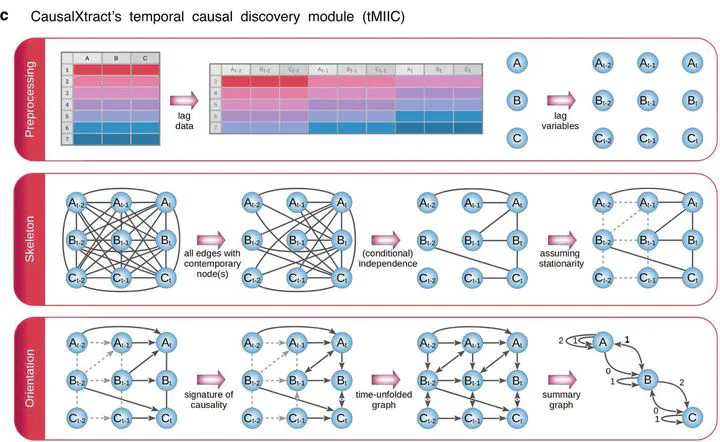
Abstract
Live-cell microscopy routinely provides massive amounts of time-lapse images of complex cellular systems under various physiological or therapeutic conditions. However, this wealth of data remains difficult to interpret in terms of causal effects. Here, we describe CausalXtract, a flexible computational pipeline that discovers causal and possibly time-lagged effects from morphodynamic features and cell–cell interactions in live-cell imaging data. CausalXtract methodology combines network-based and information-based frameworks, which is shown to discover causal effects overlooked by classical Granger and Schreiber causality approaches. We showcase the use of CausalXtract to uncover novel causal effects in a tumor-on-chip cellular ecosystem under therapeutically relevant conditions. In particular, we find that cancer-associated fibroblasts directly inhibit cancer cell apoptosis, independently from anticancer treatment. CausalXtract uncovers also multiple antagonistic effects at different time delays. Hence, CausalXtract provides a unique computational tool to interpret live-cell imaging data for a range of fundamental and translational research applications.
Type
Publication
eLife